How AI is accelerating research publishing
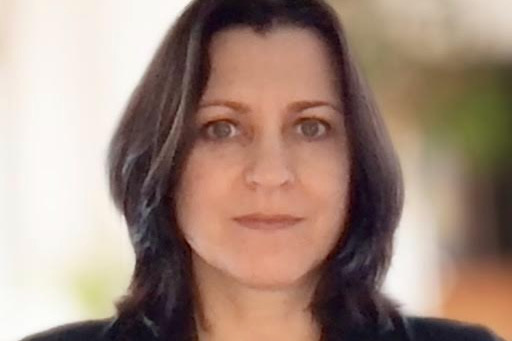
Rachel Burley
Scholarly publishing is in a state of change, and the centuries old model of traditional peer review-based publishing is under more pressure than ever to become faster and more open, writes Rachel Burley.
Covid-19 has created a greater sense of urgency to drive this change, and some academic publishers are responding by transforming their workflows and processes to reduce the editorial bottlenecks caused by growing research output coupled with limited human capacity to assess and review it.
There are several trends simultaneously leading to this pressure.
Global research output has continued to increase at a rate of about 7 per cent per year since 2012, reaching a new high of 4.7 million published articles in 2020, according to the Dimensions database. The most prestigious academic journals are selective, and acceptance criteria include ‘originality’ or ‘importance of the contribution to the field’. Acceptance rates for these journals can be as low as 5 to 10 per cent of submitted manuscripts. But an increasing number of journals accept research that is methodologically sound without the requirement for novelty, an approach introduced by mega-journals and subsequently adopted by discipline-specific titles.
This ‘low selectivity’ standard was thought to accelerate publication as reviewers are not asked to judge the importance of the research, but may have, in fact, slowed publication times by increasing the volume of eligible manuscripts. With surging submissions, the demands on the peer review community are becoming unsustainable.
More research than ever before is being published open access and approximately 30 per cent of all scholarly articles are now published as paid-for Open Access.This has changed the customer dynamic for publishers, who have transitioned from serving a relatively small customer base of institutional subscribers to a large number of individual authors paying article processing charges. This represents a transition from a B2B to a B2C model; and connecting payment with publication in this way has led to increased expectations for speed and that might not be met with traditional journal workflows.
Preprinting is becoming commonplace across research disciplines. An estimated 8 per cent of biomedical research papers were preprinted before publication in a journal in 2020, compared to 0.2 per cent in 2013. Preprints provide authors with a rapid pathway for sharing research independently of traditional publishing and with new services emerging to peer review and verify preprints, there is real potential for preprints to become the basis for an alternative publishing model.
These trends underscore the importance of improving the current academic publishing system and creating more efficient ways to assess and disseminate research results. While peer review remains a critical step in validating research results, the pandemic and the accompanying urgent need for rapid scientific advances has shone a light on the pace at which peer review moves. So, how can we make sharing research results faster and more efficient?
Artificial intelligence is now supporting authors and publications alike by speeding up the publication process while helping to preserve quality with fewer human resources, and the tools can give journals competitive advantages for attracting authors.
AI-based tools can be used in editorial processes and decision making in ways that support the often-overburdened humans who are responsible for them, and there is a growing number of working examples already supporting various stages of scholarly publishing to share.
There are now tools to help journal editors find the right peer reviewers, an increasingly difficult task that is critical in obtaining timely reviews. Two primary reasons that reviewers decline review invitations are that the article is outside their area of expertise and that they are too busy with their own research. AI can help by enabling editors to identify reviewers in specific research disciplines. Some publishers use AI for optimal human oversight, while leveraging some of the latest advancements in natural language processing and language models. Now editors can receive an unbiased ranked and ordered list of the most suitable reviewers based on the content of the paper in just a few seconds, a task that typically takes an editor 90 minutes.
Another time consuming process that journals must carry out are manuscript checks generally carried out on all submissions. Automated checks allow editors and reviewers to focus on the content of the paper rather than routine checks. Ripeta also uses natural language processing technology to target several critical elements of a scientific manuscript, including purpose, data and code availability statements; funding statements, and more to gauge the level of responsible reporting in authors’ scientific papers and suggest improvements. The service can be integrated into the journal submission process to provide data for editors and reviewers that support the peer review and decision making process.
Scholarcy is an AI-powered article summarizer that pulls out key concepts to automatically summarise papers. One use case for publishers is extracting information on data availability, study participants and analyses used, study limitations, and ethical compliance information, as part of the manuscript screening process.
AJE and its parent, Research Square Company, were built on helping to level the playing field for underrepresented researchers. The goal of our services is to ensure researchers’ works are judged on the quality of their contribution and not on their English language abilities. We began investing in AI in 2017, developing language assessment and editing tools using deep learning natural language processing technology. The technology is trained on our corpus of 900,000 edited manuscripts amassed over 15 years and is effectively supplementing the work of our own editors, improving efficiency and reducing costs. Among other things, these tools optimise our workflow by first scoring the language quality of manuscripts and then providing an automated edit, which makes an average of 1,000 changes in a 5,000-word manuscript prior to being assigned to our in-house editors.
If AI can screen manuscripts, copy edit and find peer reviewers, could it write a paper? Generative Pre-trained Transformer 3 (GPT-3) is a neural network machine learning model that produces realistic human text. In a study, professors were asked to create a writing exercise and grade submissions from both students and from GPT-3. The average time between assignment and completion for GPT-3 was three to 20 minutes compared to three days for the humans. These same professors then graded the papers, not knowing whether they came from students or the GPT-3 model. At the end of the reviews, the GTP-3 model scored an average grade of ‘C’, receiving similar feedback as the students, although professor feedback noted that it wrote shallow, less descriptive papers compared to its human counterparts.
With increasing demand for shorter publication times, there is no doubt that AI will become more commonly used in academic publishing. As the technology improves, not only can it help to increase efficiency and reduce costs in the existing research communications system; it may also have the potential to completely transform it. The importance of human judgement in the creation and evaluation of research papers is clear, but AI tools can be applied to augment tasks that humans have traditionally carried out, and they can enable greater scale and speed. As the technology improves over time, we’ll be asking how farwe should push the limits of AI.
Rachel Burley is president of Research Square